Advancing Analytics explored a use case for an international insurance company, who are part of the London insurance market, that leveraged machine learning to forecast claims volumes across all its business units, foreseeing demands three years ahead. The company forecasted using its own unique line of business segmentations to forecast a particular product.
Transforming Claims Handling with Machine Learning
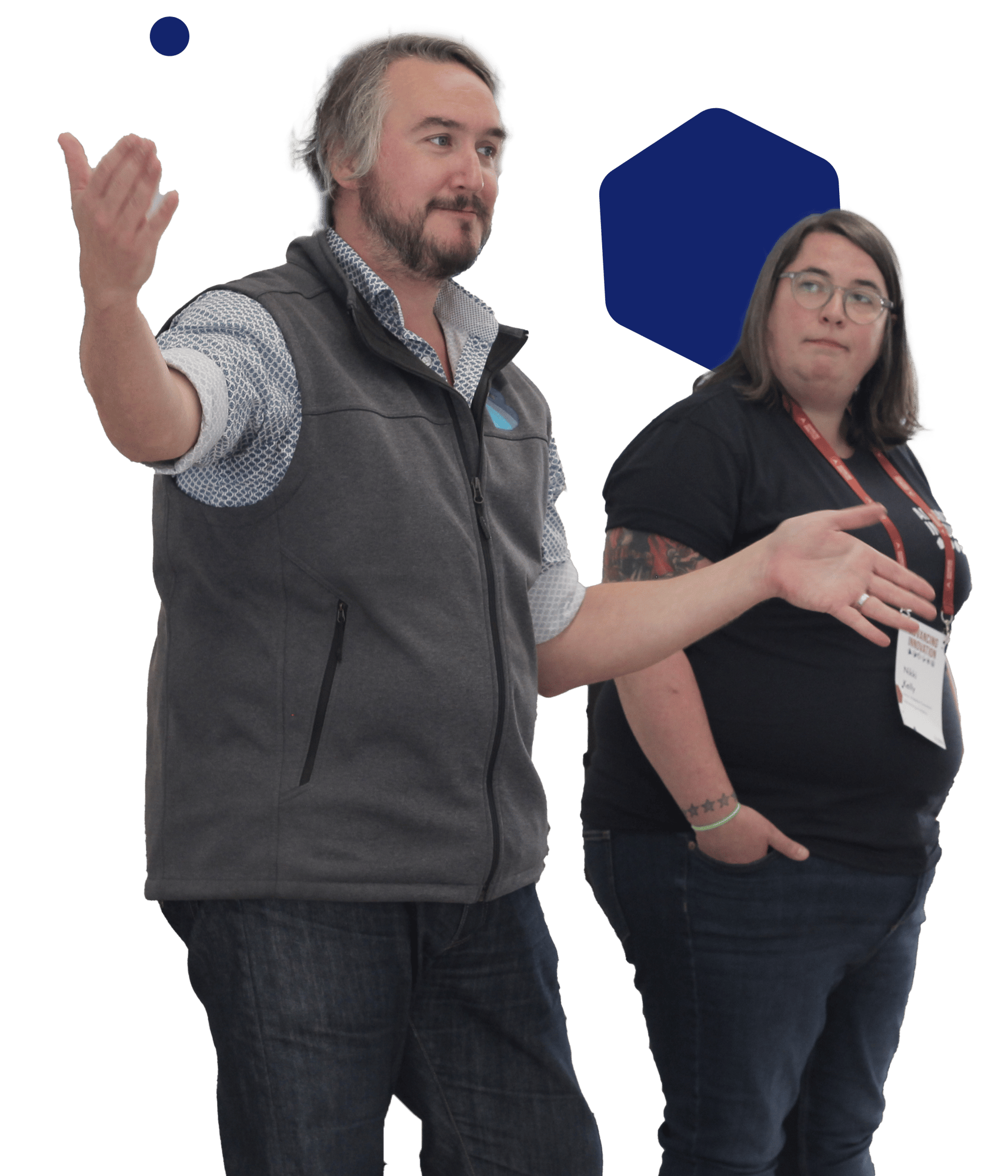
The client's challenge
In the competitive London Insurance Markets, efficient resource allocation is crucial for keeping customers happy and maintaining profits. Claims handling is a critical aspect of insurance operations, directly impacting customer experience and financial performance. The client, an international insurance conglomerate with a diverse portfolio spanning multiple geographies and insurance products, faced challenges in optimising claims handling resources. The balance between too many or too few resources can impact profitability. Firstly, an insufficient amount of handlers can result in outsourcing the claims to third parties, dramatically increasing the cost per claim. Conversely, if too many handlers are allocated, this can result in an increase in the cost per claim. The business introduced its centralised approach to the line of business segmentations. The expectation is that these segmentations will be needed for forecasting claim volumes on each segment. We had to find a solution that would address the client's specific challenges around resource allocation while allowing for their complicated operations as they were managing different types of insurance each with unique claim patterns.
.png?width=367&height=373&name=Group%201%20(2).png)
What was the solution?
To tackle these challenges, the insurance company used a data-driven approach by incorporating machine learning to forecast the need for claims handlers. The solution included:
-
Machine Learning Model: Developed a system that trains, tunes, and evaluates multiple models to predict claims volumes accurately.
-
High Accuracy: Achieved up to 92% accuracy in predictions.
-
Manual Adjustments: Allowed for manual updates to the live model’s forecasts, with changes tracked and displayed.
-
Integration: Integrated the forecasting results into the company’s resource planning systems for better allocation of claims handlers.
What were the results?
Implementing machine learning for forecasting claims handler needs brought several clear benefits:
Cost Savings: Reduced costs by optimising the number of claims handlers, avoiding expensive outsourcing.
Better Predictions: Improved accuracy in predicting claim volumes, leading to more precise resource allocation.
Competitive Edge: Gained a competitive advantage by responding quickly to market changes and customer needs.
Operational Efficiency: Streamlined operations, improving overall efficiency and customer satisfaction.
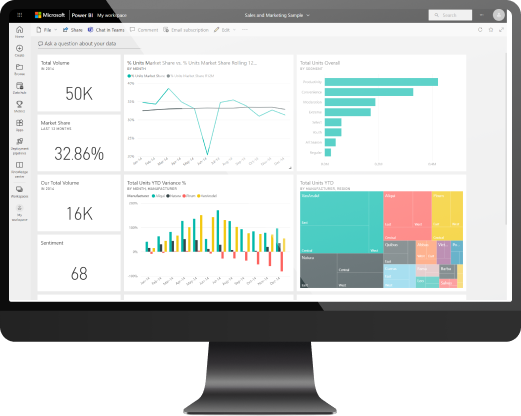
What did the client think?
.png?width=331&height=338&name=Layer_1%20(4).png)
"Advancing’s expertise and offering allowed us to select and deploy industry standard capabilities in the certainty that they were well engineered, best practice, and fit for our needs, resulting in a tailored output, rather than the roll out of a templated solution."
Product Owner for Data
London Insurance Markets client
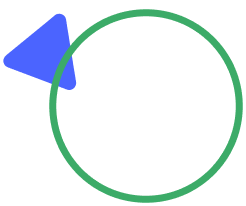
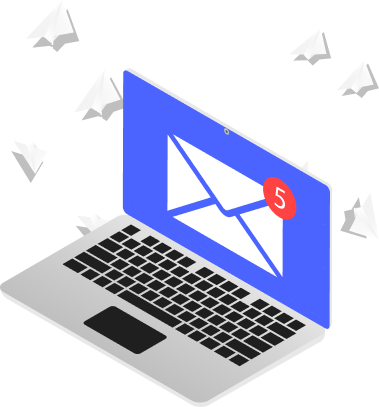