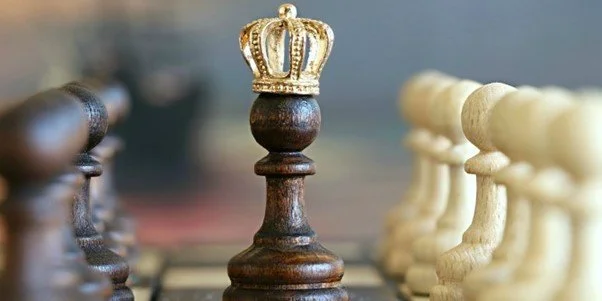
We all know the phrase "customer is king"— it's all about putting the customer at the centre of everything we do. In today's fast-paced world, customers have more options than ever before and higher expectations, so it's crucial for companies to understand and provide exceptional customer service to stay competitive. One of the best ways to do this is through customer 360 views, also known widely as a single customer view. A customer 360. This gives us a complete and unified view of the customer, including demographic data like age, gender, income, education, and occupation, as well as transactional data, behavioural data, and touch-points data. By having all this information, we can personalise interactions, improve the customer experience, and make smarter business decisions.
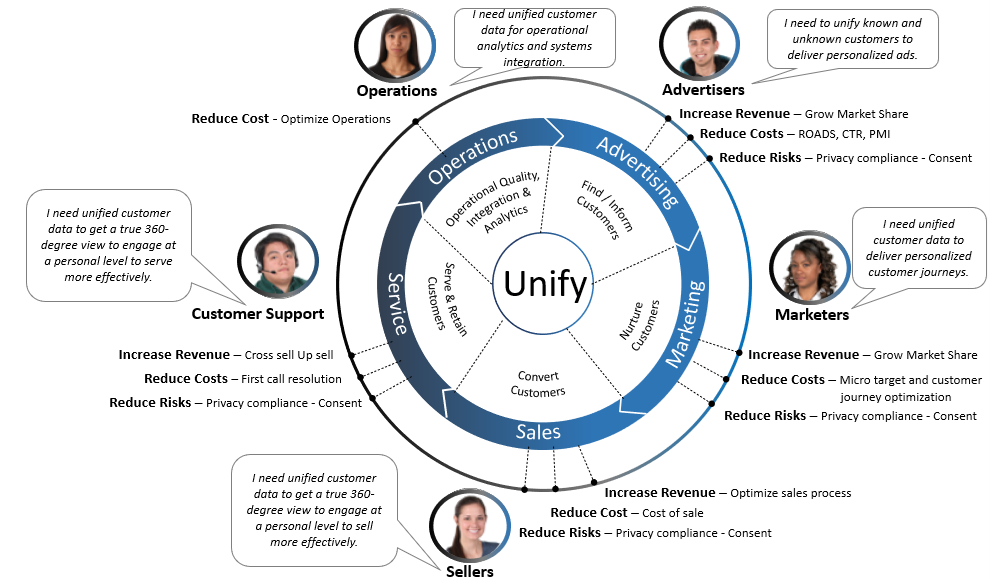
Source: Microsoft.com
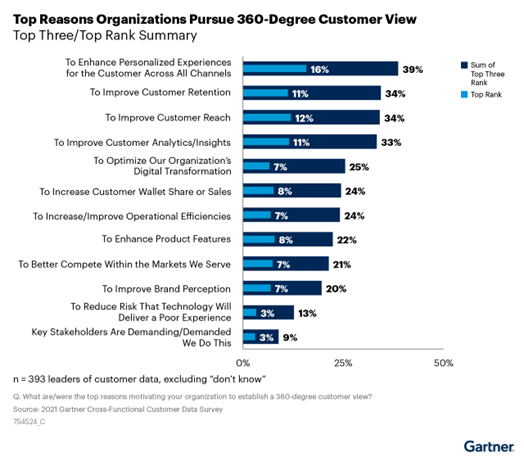
But why do organisations need a customer 360 view? Simple - it's all about having a complete and unified picture of customers. With it, businesses can make sure that they are giving their customers the best experience possible whilst making correct decisions. It's a win-win situation. A recent survey by Gartner found that the main reasons companies want a customer 360 view are for personalisation, retention, reach, and analytics.
And where is it used? Everywhere! Here are a few examples:
Retail: Retail companies use customer 360 views to gain a deeper understanding of their customers' purchasing habits and preferences. This information can be used to personalise marketing campaigns, improve the in-store experience, and increase sales through upselling and cross-selling.
Finance: Financial institutions use customer 360 views to comply with regulations such as Know Your Customer (KYC) and Anti-Money Laundering (AML) by having a complete view of their customers' financial information and transactions.
Insurance: Insurance companies use customer 360 views to track a customer's policy details, payments, claims and other interactions with the company, insurance companies can use machine learning algorithms to analyse the data and make personalised recommendations to the customer, such as additional coverage options based on the customer's specific needs and risk profile. See our recent blog on recommendation engines.
Telecommunications: Telecommunications companies use customer 360 views to gain a more complete view of their customers' usage patterns and preferences. This information can be used to personalise plans, improve customer service, and increase revenue through upselling and cross-selling.
Customer 360 can be used in many verticals or industries, but how does machine learning come into the picture? How can we use machine learning to achieve a ML-driven 360- customer view?
10 ways machine learning can be used on customer 360 view to gain more insight:
-
Personalisation: Businesses can analyse customer data from various sources, such as demographic, transactional, and behavioural data, to create personalised experiences and recommendations for each customer that are tailored to their specific needs. This can be achieved by using machine learning algorithms such as recommendation engines. Check out our articles on selecting the right recommendation algorithm and understanding recommendation engines.
-
Predictive modelling: Machine learning can be used to create predictive models that can forecast customer behaviour and preferences. For example, by analysing purchase history and browsing behaviour, a predictive model can predict which products or services a customer is likely to purchase in the future. This can be used to inform marketing and sales strategies.
-
Campaign optimisation: Machine learning can be used to optimise marketing campaigns by predicting which customers are most likely to respond to a particular campaign or offer.
-
Customer segmentation: Machine learning can be used to segment customers into different groups based on their characteristics and behaviour. By analysing customer data, machine learning algorithms can identify patterns that indicate which customers have similar needs and preferences. This allows organisations to tailor their marketing and sales efforts to specific segments. Our article on customer segmentation will demonstrate how you can jump-start your project using pre-built code designed by Databricks.
-
Fraud detection: Machine learning can be used to detect patterns of fraudulent activity in customer data. By analysing transactional data, behavioural data, and interactions data, machine learning algorithms can identify patterns that indicate fraudulent activity.
-
Risk assessment: Use machine learning to assess the risk profile of customers, which can be used to inform decisions such as credit approval or insurance underwriting.
-
Churn prediction: Machine learning can be used to identify customers who are at risk of cancelling their service or subscription. By analysing customer data, such as transactional data, behavioural data, and interactions data, machine learning algorithms can identify patterns that indicate a customer is at risk of churning. This allows organisations to take proactive measures to retain them. See our recent blog on customer retention with FLAML.
-
Natural Language Processing (NLP): Machine learning can be used to analyse customer feedback and interactions, such as survey responses or customer service transcripts, to gain insights into customer sentiment and preferences.
-
Upselling: Use machine learning to analyse customer data, such as purchase history and browsing behaviour, to make personalised product or service recommendations to increase revenue. See our recent blog here identifying upsell opportunities.
-
Image recognition: Machine learning can be used to analyse images, such as customer photos or videos, to gain insights into customer behaviour and preferences. By analysing images, machine learning algorithms can identify patterns that indicate customer preferences, such as the types of products or services they are interested in.
Challenges of developing a customer 360 platform
Developing a customer 360 platform can be challenging for several reasons. One of the main challenges is the complexity of the data. Customer data can be distributed across multiple systems and in different formats, making it difficult to integrate. Another challenge is the lack of scalability, as the data volume, velocity, and variety can grow rapidly, making it hard to handle and process the data. Also, data privacy and security are critical aspects that need to be considered when dealing with customer data, as it contains sensitive information that needs to be protected.
One solution to these challenges is to use a platform like Databricks within the Azure ecosystem. Databricks, an Apache Spark-based analytics platform that can be easily integrated with other Azure services such as Azure Data Factory, Azure Data Lake Storage, and Azure Machine Learning. This allows businesses to leverage the power of Databricks for data processing and machine learning while also taking advantage of the scalability, security, and compliance features provided by Azure.
Wrapping up
To wrap it up, using a 360 view of customers and machine learning together can be a game-changer for businesses looking to improve customer service and boost revenue. A 360 view gives a complete picture of customers, including their demographics, historical transactions, behaviour and touch-points which can be used to personalise interactions and make informed decisions. Machine learning can take it further by targeting marketing, personalising experiences, predicting customer behaviour, optimising campaigns and segmenting customers.
Topics Covered :
Author
Gavita Regunath